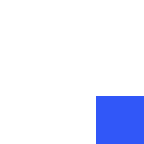
Reducing customer churn with accurate prediction models
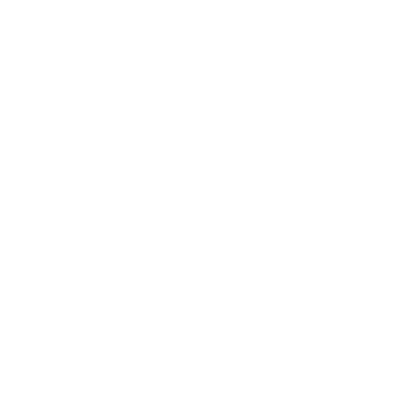
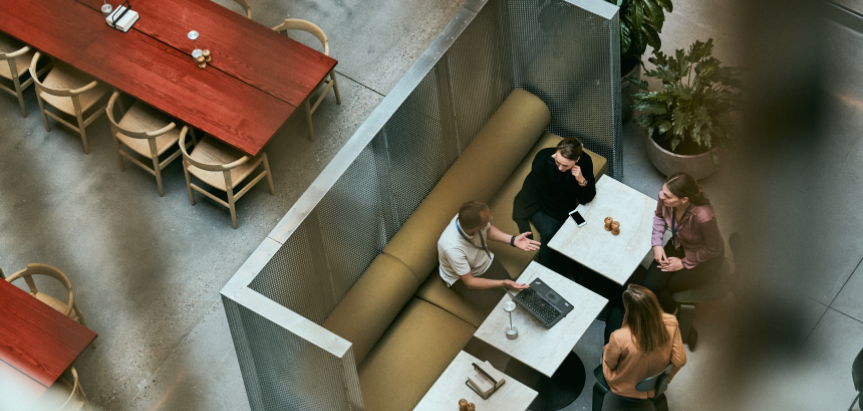
Deliverables
- 168,000 data points analysed
- Highly accurate churn prediction model
- Highly accurate sales prediction model
- New insights into churn and purchase factors
Long story short
Our accurate prediction model helped Visma Software identify customers at risk of churn and take timely action to retain them and increase sales.
About Visma
Visma is the champion of cloud software and has 1.5 million customers in 25 countries.
The marketing team at Visma wanted to identify customer behaviour before churn so that they could take timely action to prevent it. They wanted to be more precise about creating offers and identify the need for training, webinars or other services to keep customers loyal and even increase purchases.
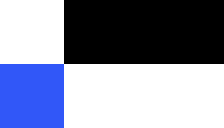
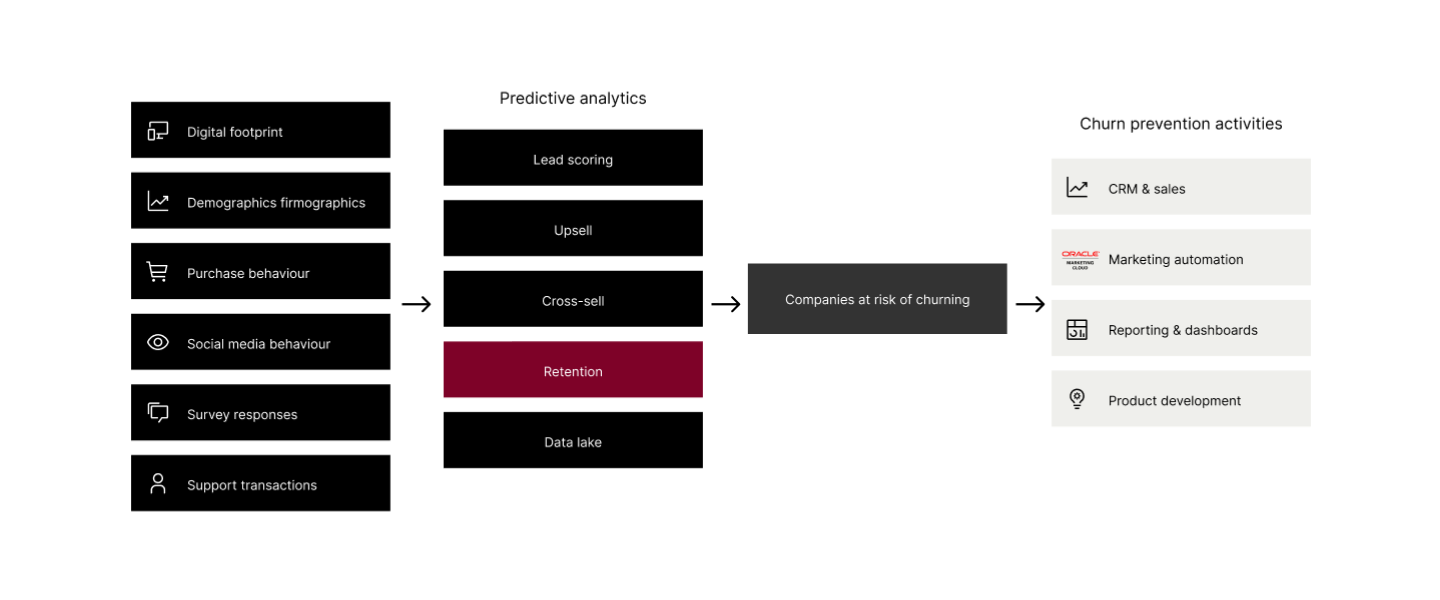
Challenge
According to a Harvard Business School report, a five per cent increase in customer retention rates results in a 25 to 95 per cent increase in profits. And yet, churn is one of the most demanding predictive analysis operations from a data requirement point of view when it comes to marketing.
This project had two main objectives. First of all, we wanted to analyse and predict the probability of both customer churn and purchases. Secondly, we wanted to get to know the factors, processes and variables that influence churn and purchase probability the most so that we could influence event outcomes effectively, either by preventing churn or accelerating purchases.
Solution
We used machine learning to predict customer churn and the likelihood of leads making a purchase of a specific service category. For the churn project we were trying to sort customers into two categories: whether they were likely to churn or not. We used 50% as a cut off, so if a customer was more than 50% likely to churn, then they were labelled as such. For the lead scoring/sales prediction project, we weren’t just trying to sort leads into two boxes. We were trying to rank them based on probability of making a purchase.
Our model combined sales and support data sets, enriched with marketing activity data and with firmographics information. All in all, we gathered data from five systems, controlled 70 variables and analysed 168,000 data points across 2,722 customer businesses for churn and an additional 6,444 potential purchasers of different service categories.
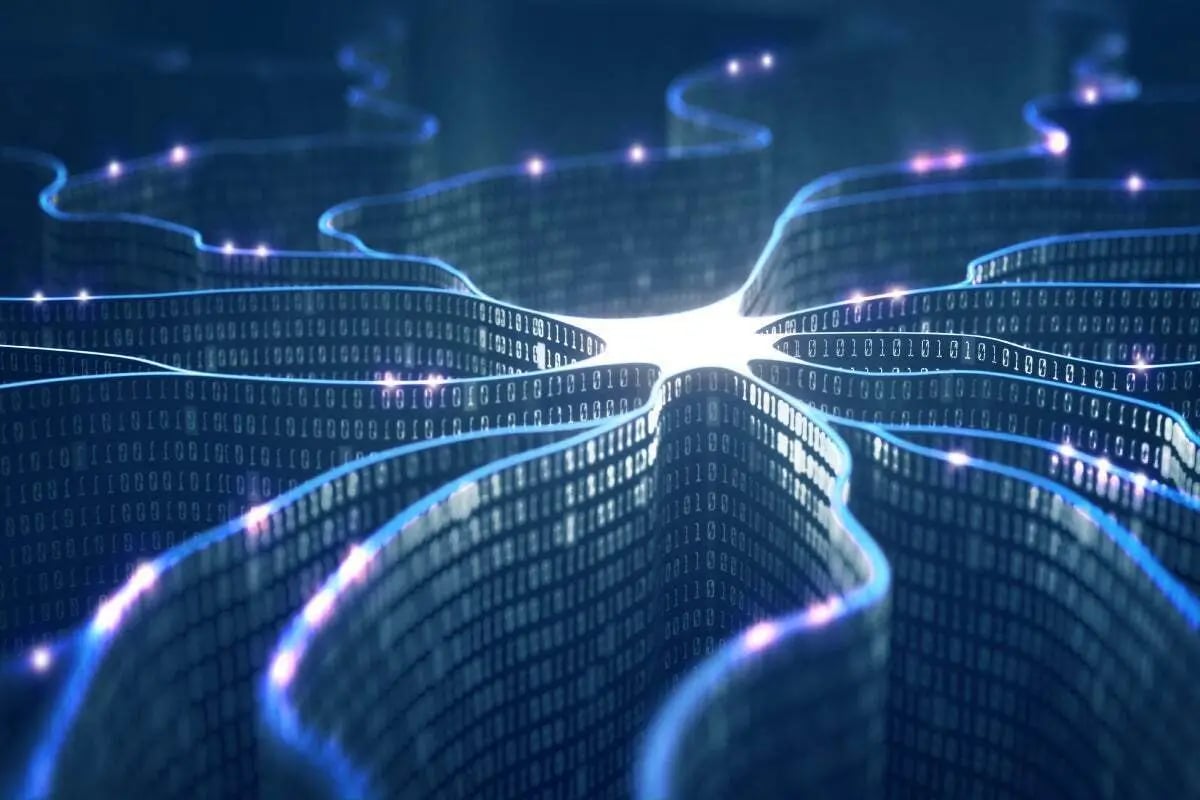
Results
Some thought it couldn’t be done, but we achieved prediction model accuracy of 84.6% for churn and 84.1% for sales—very high when it comes to predictive marketing technology. Powered by machine learning, our analysis revealed the metrics most significant for churn prevention.
By using Oracle Eloqua, the marketing team were able to harness multiple years of activity data, which helped achieve high prediction model accuracy. That empowered them team to identify 602 new leads and 722 customer businesses at risk of churn. The results also provided new insights into the processes and mechanisms that affect churn and buying.
The team is now going to implement the right tactics at the right time to increase purchases and reduce customer loss, both of which will have an effect on the bottom line for years to come.
84.6%
Model accuracy achieved for churn
84,1%
Model accuracy achieved for sales
168,000
Data points analysed (across 2,722 customer business for churn and 6,444 for potential buyers)
602
New leads identified as being at risk of churn
722
Customer businesses identified as being at risk of churn
Let's talk!
Got a hot idea or a burning challenge? Drop us a line and let's see what we can do – you lose nothing by asking.
More customer stories
Case study - AWS | kloia - Night at the Museum Case Study
Bringing witty, human storytelling to kloia’s application modernisation video marketing delivered a...
Dell - End-to-end video production
Luxid produced 30 high-quality Dell webcam videos in six languages, earning praise for...
Case study - HMD - DTC
Turning Nokia Phones' site into ecommerce success: Scalable design, Bigcommerce integration, 100%...